This is a guest post from Lubov McKone, the Industry Documents Library’s 2022 Data Science Senior Fellow.
This summer, I served as the Industry Documents Library’s Senior Data Science Fellow. A bit about me – I’m currently pursuing my MLIS at Pratt Institute with a focus in research and data, and I’m hoping to work in library data services after I graduate. I was drawn to this opportunity because I wanted to learn how libraries are using data-related techniques and technologies in practice – and specifically, how they are contextualizing these for researchers.
Project Background
The UCSF Industry Documents Library is a vast collection of resources encompassing documents, images, videos, and recordings. These materials can be studied individually, but increasingly, researchers are interested in examining trends across whole collections, or subsets of it. In this way, the Industry Documents Library is also a trove of data that can be used to uncover trends and patterns in the history of industries impacting public health. In this project, the Industry Documents Library wanted to investigate what information is lost or changed when its collections are transformed into data.
There are many ways to generate data from digital collections. In this project we focused on a combination of collections metadata and computer-generated transcripts of video files. Like all information, data is not objective but constructed. Metadata is usually entered manually and is subject to human error. Video transcripts generated by computer programs are never 100% accurate. If accuracy varies based on factors such as the age of the video or the type of event being recorded, how might this impact conclusions drawn by researchers who are treating all video transcriptions as equally accurate? What guidance can the library provide to prevent researchers from drawing inaccurate conclusions from computer-generated text?
Project Team
- Kate Tasker, Industry Documents Library Managing Archivist
- Rebecca Tang, Industry Documents Library Applications Programmer
- Geoffrey Boushey, Data Science Initiative Application Developer and Instructor
- Lubov McKone, Senior Data Science Fellow
- Lianne De Leon, Junior Data Science Fellow
- Rogelio Murillo, Junior Data Science Fellow
Project Summary
Research Questions
Based on the background and the goals of the Industry Documents Library, the project team identified the following research questions to guide the project:
- Taking into account factors such as year and runtime, how does computer transcription accuracy differ between television commercials and court proceedings?
- How might transcription accuracy impact the conclusions drawn from the data?
- What guidance can we give to researchers to prevent uninformed conclusions?
Uses
This project is a case study that evaluates the accuracy of computer-generated transcripts for videos within the Industry Documents Library’s Tobacco Collection. These findings provide a foundation for UCSF’s Industry Documents Library to create guidelines for researchers using video transcripts for text analysis. This case study also acts as a roadmap and a collection of instructional materials for similar studies to be conducted on other collections. These materials have been gathered in a public github repo, viewable here.
Sourcing the Right Data
At the beginning of the project, we worked with the Junior Fellows to determine the scope of the project. The tobacco video collection contains 5,249 videos that encompass interviews, commercials, court proceedings, press conferences, news broadcasts, and more. We wanted to narrow our scope to two categories that would illustrate potential disparities in transcript accuracy and meaning. After transcribing several videos by hand, the fellows proposed commercials and court proceedings as two categories that would suit our analysis. We felt 40 would be a reasonable sample size of videos to study, so each fellow selected 10 videos from each category, selecting videos with a range of years, quality, and runtimes. The fellows were selecting videos from a list that was generated by the InternetArchive python API, containing video links and metadata such as year and runtime.
Computer & Human Transcripts
Once the 40 videos were selected, we extracted transcripts from each URL using the Google AutoML API for transcription. We saved a copy of each computer transcription to use for the analysis, and provided another copy to the Junior Fellows, who edited them to accurately reflect the audio in the videos. We saved these copies as well for comparison to the computer-generated transcription.
Comparing Transcripts
To compare the computer and human transcripts, we conducted research on common metrics for transcript comparison. We came up with two broad categories to compare – accuracy and meaning.
To compare accuracy, we used the following metrics:
- Word Error Rate – a measure of how many insertions, deletions, and substitutions are needed to convert the computer-generated transcript into the reference transcript. We subtracted this number from 1 to get the Word Accuracy Rate (WAR).
- BLEU score – a more advanced algorithm measuring n-gram matches between the transcripts, normalized for n-gram frequency.
- Human-evaluated accuracy – a score from Poor, Fair, Good, and Excellent assigned by the fellows as they were editing the computer-generated transcripts.
- Google AutoML confidence score – a score generated by Google AutoML during transcript generation indicating how accurate Google believes its transcription to be.
To compare meaning, we used the following metrics:
- Sentiment – We generated sentiment scores and magnitude for both sets of transcripts. We wanted to see whether the computer transcripts were under- or over- estimating sentiment, and whether this differed across categories.
- Topic modeling – We ran a k-means topic model for two categories to see how closely the computer transcripts matched the pre-determined categories vs. how closely they were matched by the human transcripts
Findings & Recommendations
Relationships in the data
From an initial review of the significant correlations in the data, we gained some interesting insights. As shown in the correlation matrix, AutoML confidence score, fellow accuracy rating, and Word Accuracy Rate (WAR) are all significantly positively correlated. This means that the AutoML confidence score is a relatively good proxy for transcript accuracy. We recommend that researchers who are seeking to use computer-generated transcripts look to the AutoML confidence score to get a sense of the reliability of the computer-generated text they are working with.
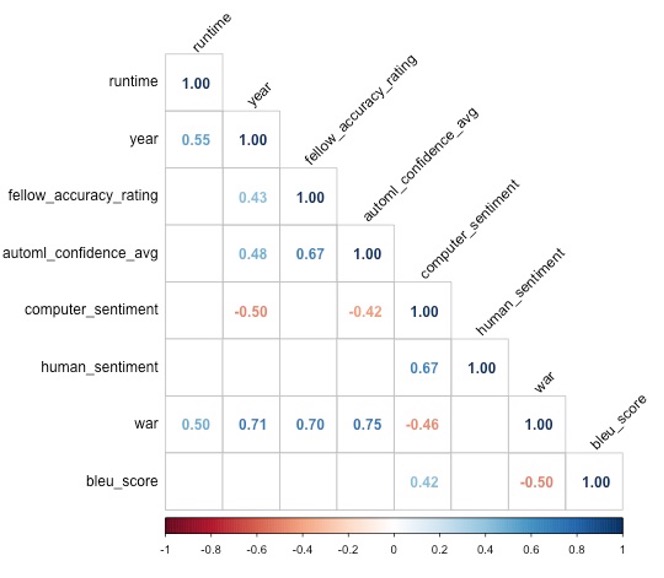
We also found a significant positive correlation between year and fellow accuracy rating, Word Accuracy Rate, and AutoML confidence score – suggesting that the more recent the video, the better the quality. We suggest informing researchers that newer videos may generate more accurate computer transcriptions.
Transcript accuracy over time
One of the Junior Fellows suggested that we look into whether there is a specific cutoff year where transcripts become more accurate. As shown in the visual below, there’s a general improvement in transcription quality after the 1960s, but not a dramatic one. Interestingly, this trend disappears when looking at each video type separately.
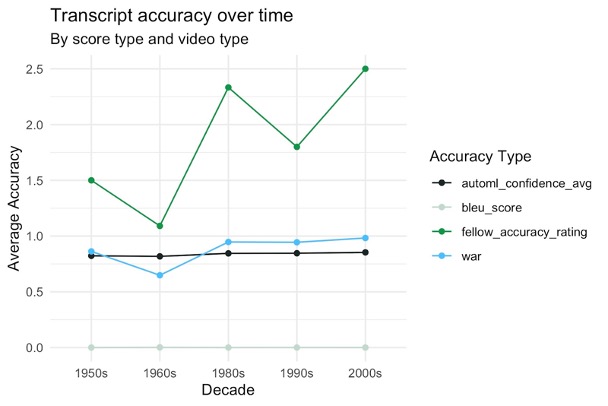
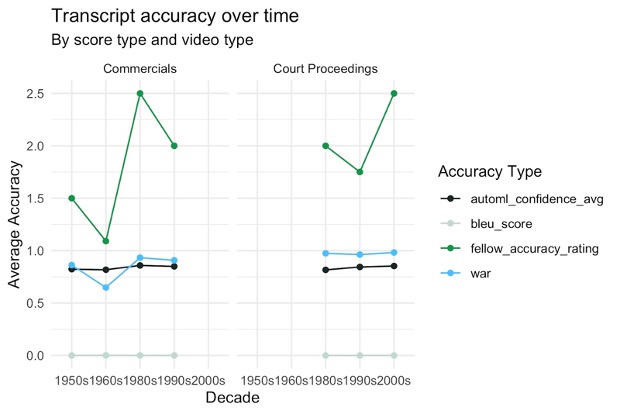
Transcript accuracy by video type
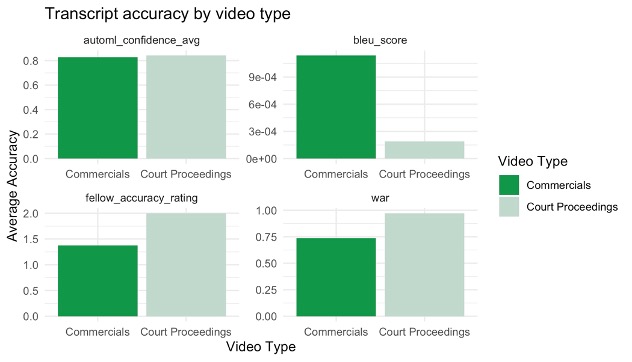
When comparing transcript accuracy between the two categories, we found that our expectations were challenged. We expected the accuracy of the advertising video transcripts to be higher, because advertisements generally have a higher production quality, and are less likely to have features like multiple people speaking over each other that could hinder transcription accuracy. However, we found that across most metrics, the court proceeding transcripts were more accurate. One potential reason for this is that commercials typically include some form of singing or more stylized speaking, which Google AutoML had trouble transcribing. We recommend informing researchers that video transcripts from media that contain singing or stylized speaking may be less accurate.
The one metric that the commercials were more accurate in was BLEU score, but this should be interpreted with caution. BLEU score is supposed to range from 0-1, but in our dataset its range was 0.0001 – 0.007. BLEU score is meant to be used on a corpus that is broken into sentences, because it works by aggregating n-gram accuracy on a sentence level, and then averaging the sentence-level accuracies across the corpus. However, the transcripts generated by Google AutoML did not contain any punctuation, so we were essentially calculating BLEU score on a corpus-length sentence for each transcript. This resulted in extremely small BLEU scores that may not be accurate or interpretable. For this reason, we don’t recommend the use of the BLEU score metric on transcripts generated by Google AutoML, or on other computer-generated transcripts that lack punctuation.
Transcript sentiment
We looked to sentiment scores to evaluate differences in meaning between the test and reference transcripts. As we expected, commercials, which are sponsored by the companies profiting off of the tobacco industry, tend to have a positive sentiment, while court proceedings, which tend to be brought against these companies, tend to have a negative sentiment. As shown in the plot to the left, the sentiment of the computer transcripts was a slight underestimation in both video types, though this was not too dramatic of an underestimation.
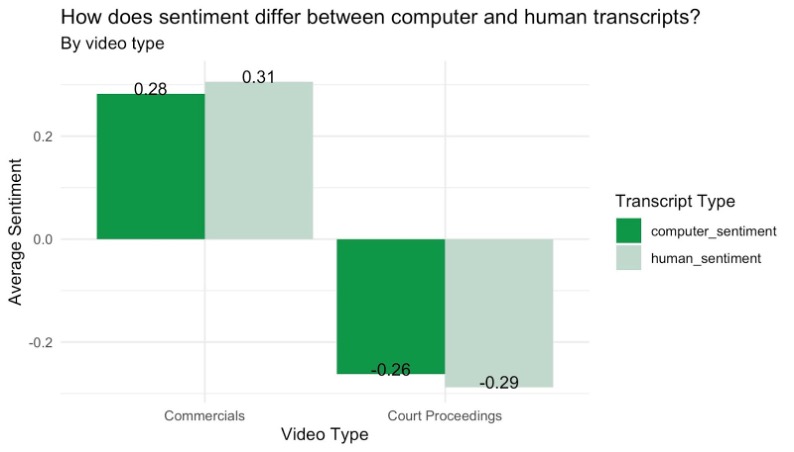
Opportunities for Further Research
Throughout this project, it was important to me to document my work and generate a research dataset that could be used by others interested in extended this work beyond my fellowship. There were many questions that we didn’t get a chance to investigate over the course of this summer, but my hope is that the work can be built upon – maybe even by a future fellow! This dataset lives in the project’s github repository under data/final_dataset.csv.
One aspect of the data that we did not investigate as much as we had hoped was topic modeling. This will likely be an important next step in assessing whether transcript meaning varies between the test and reference transcripts.
Professional Learnings & Insights
My main area of interest in the field of library data services is critical data literacy – how we as librarians can use conversations around data to build relationships and educate researchers about how data-related tools and technologies are not objective, but subject to the same pitfalls and biases as other research methods. Through my work as the Industry Documents Library Senior Data Science Fellow, I had the opportunity to work with a thoughtful team who is thinking ahead about how to responsibly guide researchers in the use of data.
Before this fellowship, I wasn’t sure exactly how opportunities to educate researchers around data would come up in a real library setting. Because I previously worked for the government, I tended to imagine researchers sourcing data from government open data portals such as NYCOpenData, or other public data sources. This fellowship opened my eyes to how often researchers might be using library collections themselves as data, and to the unique challenges and opportunities that can arise when contextualizing this “internal” data for researchers. As the collecting institution, you might have more information about why data is structured the way it is – for instance, the Industry Documents Library created the taxonomy for the archive’s “Topic” field. However, you are also often relying on hosting systems that you don’t have full control over. In the case of this project, there were several quirks of the Internet Archive API that made data analysis more complicated – for example, the video names and identifiers don’t always match. I can see how researchers might be confused about what the library does and does not have control over.
Another great aspect of this fellowship was the opportunity to work with our high school Junior Fellows, who were both exceptional to work with. Not only did they contribute the foundational work of editing our computer-generated transcripts – tedious and detail-oriented work – they also had really fresh insights about what we should analyze and what we should consider about the data. It was a highlight to support them and learn from them.
I also appreciated the opportunity to work with this very unique and important collection. Seeing the breadth of what is contained in the Industry Documents Library opened my eyes to not only the wealth of government information that exists outside of government entities, but also to the range of private sector information that ought to be accessible to the public. It’s amazing that an archive like the Industry Documents Library is also so invested in thinking critically about the technical tools that it’s reliant upon, but I guess it’s not such a surprise! Thanks to the whole team and to UCSF for a great summer fellowship experience!